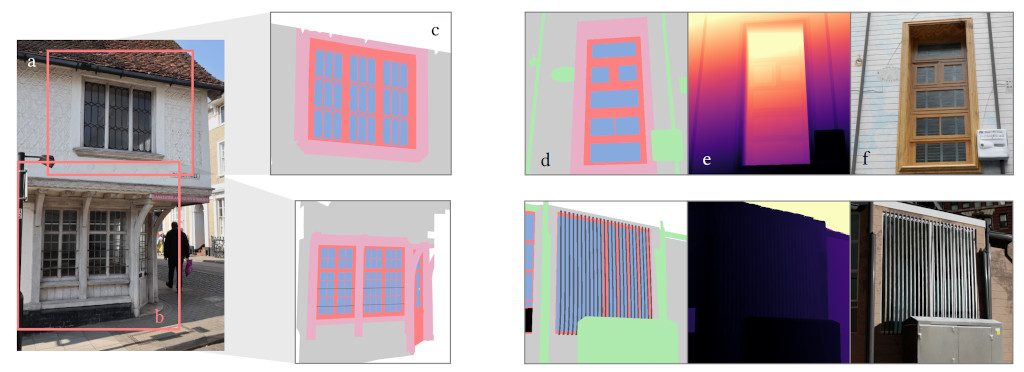
Abstract
We present WinSyn, a unique dataset and testbed for creating high-quality synthetic data with procedural modeling techniques. The dataset contains high-resolution photographs of windows, selected from locations around the world, with 89,318 individual window crops showcasing diverse geometric and material characteristics. We evaluate a procedural model by training semantic segmentation networks on both synthetic and real images and then comparing their performances on a shared test set of real images. Specifically, we measure the difference in mean Intersection over Union (mIoU) and determine the effective number of real images to match synthetic data’s training performance. We design a baseline procedural model as a benchmark and provide 21,290 synthetically generated images. By tuning the procedural model, key factors are identified which significantly influence the model’s fidelity in replicating real-world scenarios. Importantly, we highlight the challenge of procedural modeling using current techniques, especially in their ability to replicate the spatial semantics of real-world scenarios. This insight is critical because of the potential of procedural models to bridge to hidden scene aspects such as depth, reflectivity, material properties, and lighting conditions.
Resources
- Supplemental (100Mb)
- Explorable dataset website
- Pre-cooked datasets
- Access the meta and raw data
- Synthetic model
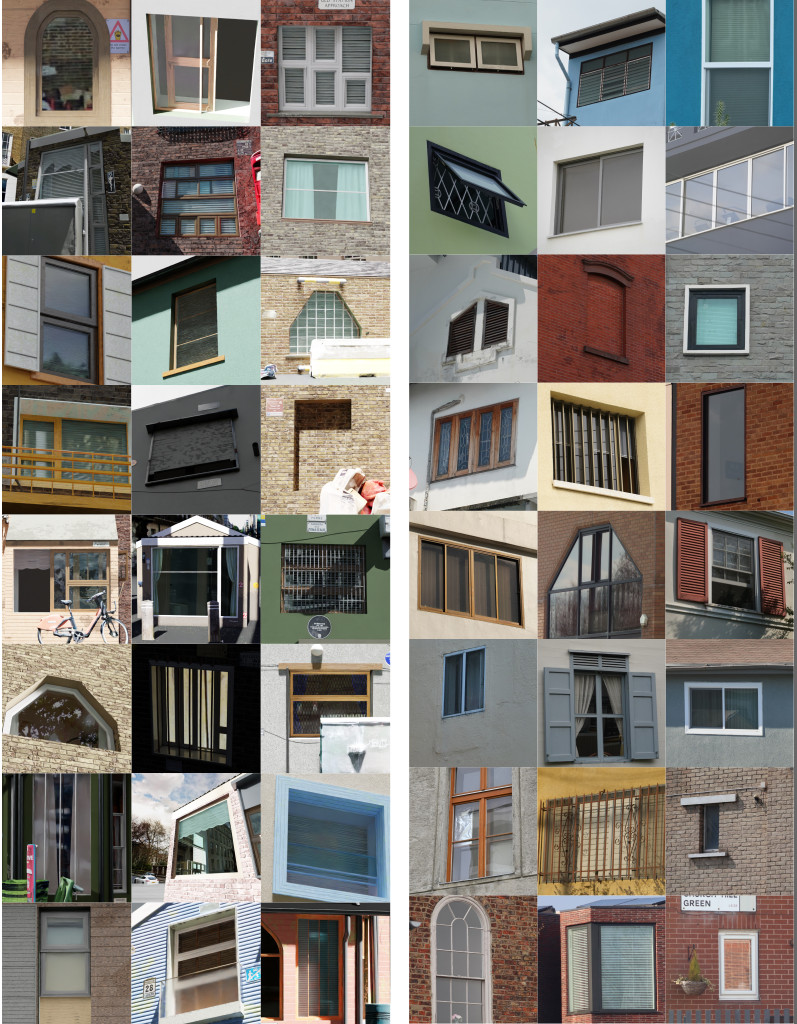
Selected real and synthetic/procedural examples from the testbed.
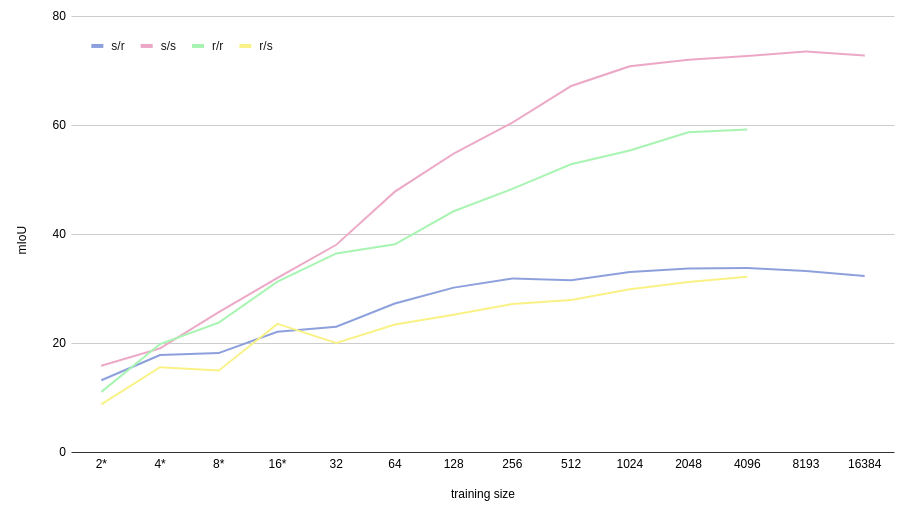
WinSyn has an appropriate level of complexity to study the synthetic data problem in the lab. Here we see the segmentation task axis (mIoU) for different and sizes of training data. s/r trains on synthetic with varying numbers samples, and evaluates on 4.9k real.
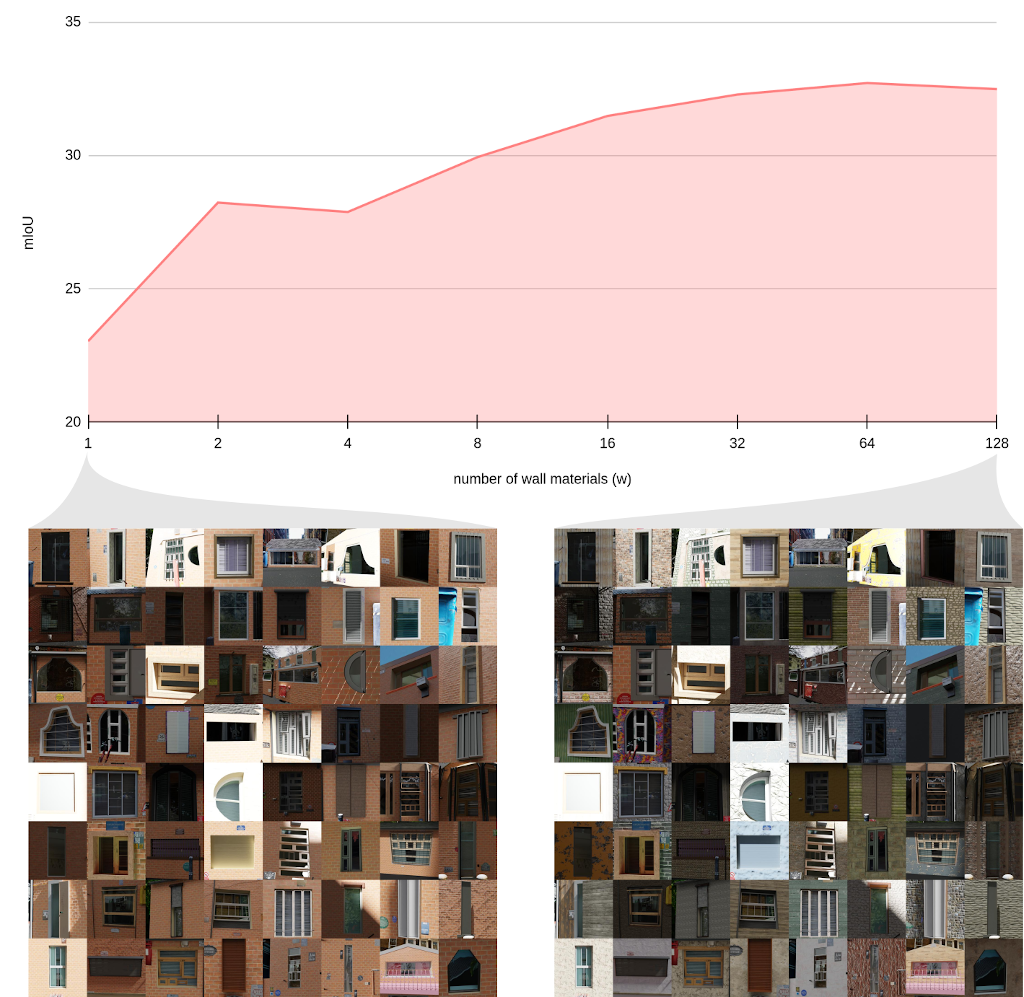
We are also able to consider a large number of synthetic model variations. Top: an experiment with the impact of the number materials available for the wall geometry (n) on segmentation task performance (mIoU). Bottom: dataset examples for n=1 (left) and n=128 (right).
A video showing a (non-final) model labelling results (worst first, best last):
BibTeX
@article{kelly2023winsyn, title = {WinSyn: A High Resolution Testbed for Synthetic Data}, author = {Tom Kelly and John Femiani and Peter Wonka}, url = {https://twak.github.io/winsyn/ https://github.com/twak/winsyn_metadata https://github.com/twak/winsyn}, year = {2024}, date = {2024-03-01}, journal = {Proceedings of the IEEE/CVF Conference on Computer Vision and Pattern Recognition}, }
Acknowledgements
We would like to thank our lead photographers Michaela Nömayr and Florian Rist, and engineer Prem Chedella, as well as our contributing photographers: Aleksandr Aleshkin, Angela Markoska, Artur Oliveira, Brian Benton, Chris West, Christopher Byrne, Elsayed Saafan, Florian Rist, George Iliin, Ignacio De Barrio, Jan Cuales, Kaitlyn Jackson, Kalina Mondzholovska, Kubra Ayse Guzel, Lukas Bornheim, Maria Jose Balestena, Michaela Nömayr, Mihai-Alexandru Filoneanu, Mokhtari Sid Ahmed Salim, Mussa Ubapa, Nestor Angulo Caballero, Nicklaus Suarez, Peter Fountain, Prem Chedella, Samantha Martucci, Sarabjot Singh, Scarlette Li, Serhii Malov, Simon R. A. Kelly, Stephanie Foden, Surafel Sunara, Tadiyos Shala, Susana Gomez, Vasileios Notis, Yuan Yuan, and LYD for the labeling. Last, but not least, we also thank the blender procedural artists Gabriel de Laubier for the UCP Wood material and Simon Thommes for the fantastic Br’cks material. Both were modified and used in our procedural model.